Data analysis, a practice rooted in history dating back centuries, had its origins with the inception of Business Intelligence (BI) in the 1800s. Data analysis has undergone a profound evolution, driven by the advent of new technologies and innovative methods for structuring, disassembling, evaluating, and safeguarding actionable insights derived from data. The worldwide predictive analytics market is currently experiencing a Compound Annual Growth Rate (CAGR) of approximately 24.5% and is poised to achieve a valuation of around $22.1 billion by the year 2026. In this transformative journey, Machine Learning (ML) has emerged as a pivotal catalyst. Machine Learning has assumed a central role in reshaping these advancements, effectively automating the data analysis process and optimizing workflows to yield deeper, comprehensive insights.
Top ML Approaches for Data Analytics
Clustering
Clustering, an advanced ML approach, involves categorizing datasets into groups where similar unlabeled data points are grouped together. Dissimilar data points are placed in separate groups. This method structures similar and dissimilar datasets, providing a clearer understanding of business processes. While many business intelligence tools can group data, ML-driven clustering allows for customized processing of large datasets, offering a cost-effective analytics solution. This approach finds applications in areas like customer segmentation and medical imaging.
Predictive Analytics
Predictive analytics, an advanced analytical method, relies on historical data to forecast future outcomes. It employs data mining techniques and ML to create statistical models that identify patterns, associations, and correlations. This approach is particularly valuable for addressing business challenges and answering questions. Unlike standard business intelligence tools, predictive analytics using ML offers customization and is suitable for various industries, including eCommerce, banking, insurance, and more. It helps with tasks such as forecasting sales, risks, and financial modeling.
Anomaly Detection
Anomaly detection is a technique for identifying rare or suspicious events or observations within datasets. These anomalies stand out when subjected to statistical analysis and differ from the rest of the data. ML-powered anomaly detection automatically processes large datasets and filters out anomalies, a task that is often overlooked by typical analytical tools. This approach is beneficial in industries such as banking, financial institutions, manufacturing, and healthcare for quickly identifying issues like fraudulent activities, defective products, or inconsistencies.
Explainable AI
Explainable AI represents the next stage in artificial intelligence and machine learning. It aims to provide autonomous systems with the ability to explain their decisions and actions. However, this technology faces challenges due to machine limitations in providing explanations. Explainable AI has the potential to transform various industries, including healthcare, transportation, manufacturing, and banking. It enables machines to explain their decision-making processes, making it invaluable in critical applications like predictive maintenance and climate change modeling.
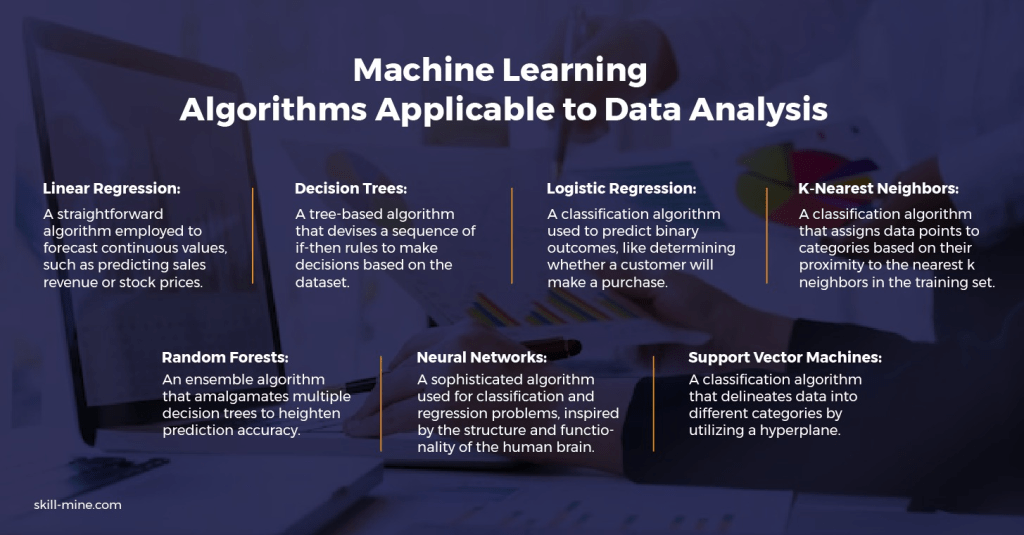
Conclusion
In numerous ways, data analysis serves as a foundation and a supplementary component to Machine Learning. By incorporating ML methodologies, data analysts can mechanize repetitive processes, enhance their grasp of data, employ algorithms to validate hypotheses, bolster predictive capabilities, manage risks, and ultimately contribute to more robust recommendations and corporate decision-making. Skillmine’s data analysis and visualization solution, Data V, empowers businesses with in-depth insights through robust analytics and visually compelling representations.
Looking for expert technology consulting services? Contact us today.